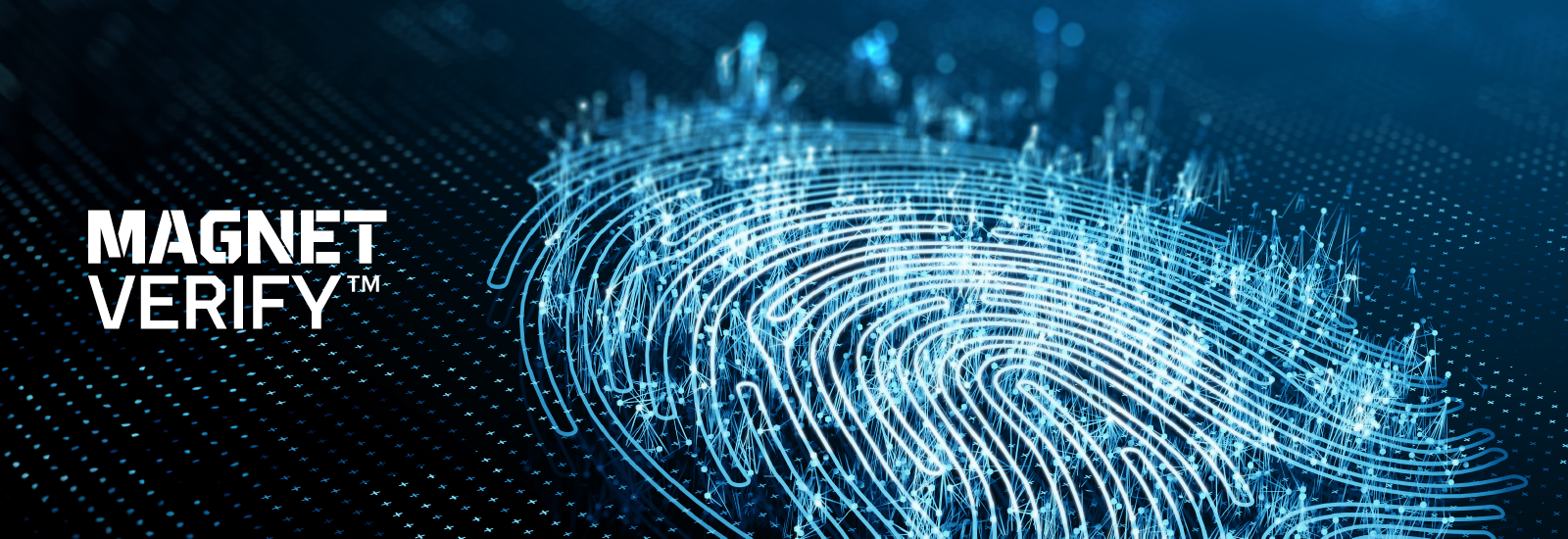
Evaluating the use of AI in digital evidence and courtroom admissibility
The use of AI in criminal, civil, and other investigations continues to grow. However, unlike other applications, employing AI in digital forensics has different considerations when it comes to admissibility in legal proceedings. These considerations stem from the gravity of the questions being answered by AI in digital forensics—questions that could affect people’s liberty, livelihoods, and finances. It is imperative that any AI applied in digital evidence analysis be met with the same rigor as other forms of forensic evidence. Implementation of AI tools developed without consideration of legal admissibility has a potential for disastrous results, whether evidence is deemed inadmissible, or worse, incorrectly analyzed.
What is “Explainable AI”?
“Explainable AI” is on track to be the next buzzword in the use of AI in digital forensics, from the detection of synthetic or deepfake media to the analysis of large amounts of data. It is expected that a hallmark of any AI tool used to produce admissible evidence be Explainable AI. To be considered Explainable AI, the tool, and underlying algorithm, needs to meet the following four principles as defined by NIST Circular 8312, “Four Principles of Explainable Artificial Intelligence.”1
- Explanation: Systems deliver accompanying evidence or reason(s) for all outputs
- Meaningful: Systems provide explanations that are understandable to individual users
- Explanation Accuracy: The explanation correctly reflects the system’s process for generating the output
- Knowledge Limits: The system only operates under conditions for which it was designed or when the system reaches a sufficient confidence in its output
Based on these principles, it is imperative any AI tool be transparent in reporting results and do so in such a way that the end user can adequately understand (and explain) those results. In terms of synthetic media and deepfake detection, this means that a simple yes or no response from a submitted video, or a bounding box around a face with a green light or red light is not sufficient in explaining how the algorithm reached a result. The question of “why” must be included in the response—why did the algorithm say yes/no to this specific video, and with how much confidence in the response? Confidence in findings is also more than a numerical value or percentage noted in a result. Context must be given to that confidence rating so the end user actually understands what the value means and, in turn, can accurately express that level of confidence in reporting and testimony. Without the context of an explanation and confidence in findings, the result is not sufficiently explainable.
Explainable AI and the federal rules of evidence
The authentication of digital evidence in the United States is largely governed by FRE 901(a) which states, “...the proponent must produce evidence sufficient to support a finding that the item is what the proponent claims it is.”2 Demonstrating that the evidence is what it purports to be can be accomplished in a variety of ways, most often through witness testimony, i.e., “I wrote that document,” or “It is me in that video,” and the offered evidence is in fact what it purports to be. Being that the AI tool or algorithm cannot testify in court to authenticate generated results, FRE 901(b)(9) would apply, satisfying the authentication rule by describing a process or system and showing that it produces an accurate result. This means a witness with knowledge of the AI tool could provide (likely) expert testimony that could authenticate the results and admit evidence in court.
The premise of “Explainable AI” is critical to fulfill the FRE pertaining to authenticating evidence when the witness would need to describe the process and show that it produced an accurate result. This may seem like a daunting task as numerous subject matter experts—as well as computer and data scientists—collaborate on the development of complex AI algorithms, explainable or not. This is where FRE 602 provides a respite from overcomplicating the introduction of evidence by eliciting testimony from numerous experts that developed an AI tool. FRE 602 requires that the authenticating witness have personal knowledge of how the AI technology functions. It states, “A witness may testify to a matter only if evidence is introduced sufficient to support a finding that the witness has personal knowledge of the matter.” This means the end user of an AI-enabled, digital forensics tool is capable of introducing the results of that tool, explaining the process (at a high level) and articulating that it produced an accurate result. Again, the ability to explain the process is critical to the authentication or introduction of evidence.
Application of Daubert to explainable AI
The U.S. federal court system, as well as most states, relies upon the Daubert Standard3 for the introduction of new scientific testimony in court. Since AI-enabled digital forensics tools are the cutting edge of technology, the introduction of results from those tools are sure to be evaluated against Daubert in order to prevent “junk science” from entering the courtroom. That evaluation is based upon five tenets identified in the Daubert standard:
- Whether the technique or theory in question can be, and has been, tested
- Whether it has been subjected to publication and peer review
- Its known or potential error rate
- The existence and maintenance of standards controlling its operation
- Whether it has attracted widespread acceptance within a relevant scientific community
In evaluating the first, and arguably most important question in a Daubert analysis, the underlying algorithm employed in an AI digital forensics tool must be explainable to effectively determine “whether the technique or theory in question can be, and has been, tested.”
The importance of transparency in AI-enabled tools is highlighted in the Northwestern Journal of Technology and Intellectual Property4 where the Honorable Paul Grimm, Dr. Maura Grossman, and Dr. Gordon Cormack noted, “…when the validity and reliability of the system or process that produces AI evidence has not properly been tested, when its underlying methodology has been treated as a trade secret by its developer preventing it from being verified by others…or when the methodology is not accepted as reliable by others in the same field, then it is hard to maintain with a straight face that it does what its proponent claims it does, which ought to render it inauthentic and inadmissible.” Simply put, if an AI-enabled tool does not disclose how it came to a result in the way an end user can explain in court, the use of that tool may be inadmissible in legal proceedings.
Magnet Verify, “Explainable AI” and legal admissibility
Magnet Verify is a forensically sound tool which produces results that are accurate, repeatable, and acceptable for use in court. Verify employs multiple approaches to file format analysis, including a deterministic structural analysis (one-to-one comparison and match) and a probabilistic attribute similarity analysis (identification of a single brand/model) based on a machine learning algorithm. We can apply the Four Principles of Explainable Artificial Intelligence to a Magnet Verify output as follows:
- Explainable: Systems deliver accompanying evidence or reason(s) for all outputs. Magnet Verify reports multiple data points in response to file analysis, including the structural signature, attribute similarity analysis, proprietary structural data, and structural consistency analysis. Verify was built to examine and report on every bit of data (no pun intended) within a video file. All of that data is presented to the end user for them to make an informed decision about file authenticity and provenance. There is no hidden interpretation of data, and Verify clearly defines all sources of results from an analysis.
- Meaningful: Systems provide explanations that are understandable to individual users. While results are displayed for the user, it is critical for the user to properly interpret those results. Magnet Verify offers various training courses for users to properly understand all results, articulate findings in reports, and be able to give testimony in court on their findings. With the addition of Verify Insights, it’s even easier for the end user to understand results as specific indicators related to the reasoning behind a Verify authenticity and provenance analysis are included with results.
- Explanation Accuracy: The explanation correctly reflects the system’s process for generating the output. A core aspect of Magnet Verify training is the explanation of what the tool is doing to produce an output and how to verify results in individual examinations. Verify also regularly tests its Attribute Similarity Analysis AI and publishes the results for users.
- Knowledge Limits: The system only operates under conditions for which it was designed, or when the system reaches a sufficient confidence in its output. Verify’s Attribute Similarity Analysis AI will only return a result when there is 90% or higher confidence in the result. Even when a result is returned, users receive training on how to verify and build confidence in the result to ensure accuracy.
As a tool purpose-built for forensic analysis of digital video files, accuracy and explainability is of paramount importance in Magnet Verify. This is evident not only in the compliance with the guiding principles of Explainable AI, but the specific considerations within the Verify tool and training to ensure that results can be used effectively in court.
For more information about Magnet Verify and courtroom admissibility, check out the product page here.
_____________________________
1 https://doi.org/10.6028/NIST.IR.8312
2 While the Federal Rules of Evidence (FRE) is applicable to cases in federal jurisdiction, the vast majority of states’ rules of evidence mirror the FRE.
3 Daubert v. Merrell Dow Pharmaceuticals, Inc., 509 U.S. 579 (1993).
4 Paul W. Grimm, Maura R. Grossman, and Gordon V. Cormack, Artificial Intelligence as Evidence, 19 NW. J. TECH. & INTELL. PROP. 9 (2021). https://scholarlycommons.law.northwestern.edu/njtip/vol19/iss1/2